Modeling Commute Behavior Dynamics in Response to Policy Changes: A Case Study from the COVID-19 Pandemic
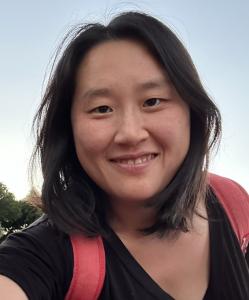
This dissertation introduces a novel model for integration into an Agent-Based Model (ABM) framework, aimed at estimating and predicting workers' commuting behaviors in a post-pandemic context. The model is designed to inform policy-making by analyzing commuting patterns and their responsiveness to policy changes. The methodology involves three stages: first, identifying worker commuting preference classes through latent class analysis; second, predicting class membership based on demographic features; and third, estimating commuter types (commuter, hybrid commuter, or telecommuter) using logistic regression. The study utilizes the ASU Covid Future Panel Survey data to identify diverse worker classes with distinct telecommuting experiences and preferences. Key findings include the discovery of various worker classes, such as regular commuters and telecommuters, and a class inclined towards high-frequency telecommuting under supportive policies. The research also explores intra-class commuter type estimation and factors influencing commuting choices, offering valuable insights for future commuting pattern predictions and policy development.